Revolutionizing AI Training: The Rise of Synthetic Imagery Unleashing New Frontiers
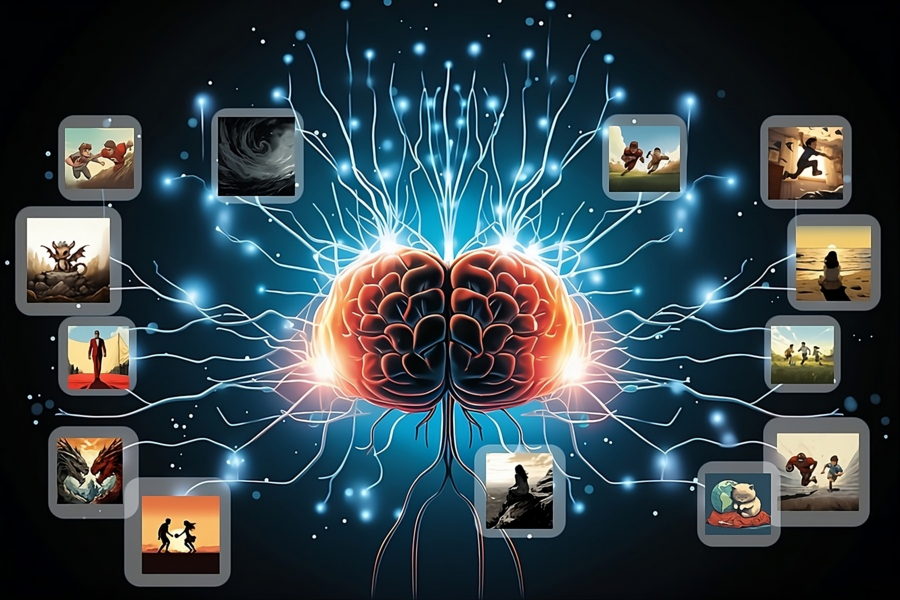
In a groundbreaking development, MIT's Computer Science and Artificial Intelligence Laboratory (CSAIL) is reshaping the landscape of artificial intelligence (AI) through the use of synthetic imagery. Led by MIT PhD student Lijie Fan and his team, this innovative approach promises to enhance training efficiency and reduce biases in machine learning, ushering in a new era of possibilities.
The Core Innovation: StableRep and Multi-Positive Contrastive Learning
At the heart of this transformative methodology is the StableRep system, a departure from traditional real-image datasets. StableRep leverages synthetic images generated by state-of-the-art text-to-image models like Stable Diffusion. The key to its success lies in "multi-positive contrastive learning," a strategy that propels the model beyond conventional data feeding by providing richer information during training.
Surpassing Real-Image Models: A Leap Forward in AI Training
StableRep's approach of treating multiple synthetic images from the same text as positive pairs has yielded remarkable results. Outshining top-tier models like SimCLR and CLIP on extensive datasets, StableRep not only mitigates data acquisition challenges but also paves the way for a new era of AI training techniques.
Fine-Tuning for Success: The Guidance Scale and Synthetic Image Superiority
A pivotal achievement of StableRep is the fine-tuning of the "guidance scale" in the generative model. This delicate adjustment ensures a balance between synthetic images' diversity and fidelity, positioning them as equally effective, if not more so, than real images in training self-supervised models.
Language Supervision Unleashed: Introducing StableRep+
Taking the innovation a step further, the team introduces language supervision in StableRep+, demonstrating superior accuracy and efficiency. Trained with 20 million synthetic images, StableRep+ surpasses CLIP models trained with 50 million real images, showcasing the potential of synthetic imagery in large-scale AI training.
Challenges on the Horizon: Addressing Limitations and Concerns
Despite its successes, the journey towards AI utopia is not without challenges. The team candidly addresses issues such as the slow pace of image generation, semantic mismatches, biases, and complexities in image attribution. While StableRep reduces dependency on vast real-image collections, concerns about hidden biases in uncurated data persist.
Balancing Act: Navigating Biases in Synthetic Imagery
The debate over biases within uncurated data used for text-to-image models takes center stage. Fan underscores the need for meticulous text selection or human curation to address potential biases in the synthesis process. While synthetic imagery offers efficiency, concerns about hidden biases emphasize the ongoing need for improvements in data quality and synthesis.
Implications Beyond Academia: A Reality Check for Generative Model Learning
The significance of this research extends beyond academia, as noted by Google DeepMind researcher David Fleet. The team's presentation of StableRep at the 2023 Conference on Neural Information Processing Systems (NeurIPS) in New Orleans marks a pivotal moment in AI advancement, promising a future where synthetic imagery sets the new standard for efficiency and bias reduction in machine learning.
Conclusion
MIT's pioneering use of synthetic imagery in AI training, as exemplified by StableRep, signifies a monumental leap forward in the realm of machine learning. The success of multi-positive contrastive learning and fine-tuning the guidance scale underscores the potency of synthetic images, outperforming traditional real-image models.
While challenges like slow image generation and potential biases persist, the presented advancements herald a future where the efficiency and versatility of synthetic imagery redefine the landscape of AI training. As we stand at the cusp of this technological revolution, the ongoing pursuit of data quality improvements and ethical considerations remains crucial for realizing the full potential of synthetic imagery in shaping the future of artificial intelligence.